Mingde Optoelectronics Artificial Intelligence Sorting Machine take the lead in introducing artificial intelligence methods such as deep convolutional neural networks(CNN) in the field of visible light photoelectic sorting to analyze and process material images,and through CNN partial connection,weight sharing,multiple convolution kernels and other methods,during the training process, the multi-dimensional features of materials are automatically extracted to establish a database,whose sorting effect is far better than traditional photoelectric methods.
Technical Advantages
1,Introduced artifical intelligence methods such as deep convolutional neural networks(CNN) in the field of visible light photoelectric sorting to analyze and process material images.
2,AI photoelectric sorting technology can automatically extract the multi-dimensional characteristics of materials,like texture,shape,color,quality,luster,etc., which greatly improves the sorting effect,expands the sorting scene and material types,to meets the market diversification and personalized sorting requirements,and solves the problem of limited color sorting materials in current color sorter market.
3,Photoelectric sorting requires high real-time performance,while CNN operation is relatively slow.In this regard, we adopt the model compression technology to accelerate CNN operation speed and greatly improve the recognition efficiency.
4,In view of the situation that many mineral materials cannot obtain massive data,our company adopts transfer lerning technology and industrial image sample enhancement technology to ensure the recognition accuracy of non-massive data training.
5, The AI sorting machine uses a gigabit camera to transmit image data to multi-GPU computing platform,which adopts CNN to analyze material types and accurately identify material surface features and texture structures.
Model
|
Mineral particle size (cm)
|
Capacity
(T/H)
|
Air pressure (Mpa)
|
Sorting accuracy
|
Optimized Carryover ratio
|
Power(kW)
|
Dimension(mm)
|
Weight(kg)
|
MAI-D4
|
3<d≤6
|
30~45
|
0.55
|
96
|
10:1
|
4.5
|
4000*2650*1760
|
2100
|
1<d≤3
|
10~12
|
0.55
|
0.5≤d≤1
|
6~8
|
0.5
|
MAI-D5
|
3<d≤6
|
38~52
|
0.55
|
96
|
10:1
|
5.0
|
4000*3160*1760
|
2250
|
1<d≤3
|
12.5~15
|
0.55
|
0.5≤d≤1
|
8~10
|
0.5
|
MAI-D6
|
3<d≤6
|
45~68
|
0.55
|
96
|
10:1
|
5.5
|
4000*3670*1760
|
2350
|
1<d≤3
|
15~18
|
0.55
|
0.5≤d≤1
|
9~12
|
0.5
|
MAI-S4
|
3<d≤6
|
35~50
|
0.55
|
99.8
|
20:1
|
6.0
|
4850*2650*2750
|
3000
|
1<d≤3
|
12~14
|
0.55
|
0.5≤d≤1
|
7~9
|
0.5
|
MAI-S5
|
3<d≤6
|
44~60
|
0.55
|
99.8
|
20:1
|
6.5
|
4850*3160*2750
|
3250
|
1<d≤3
|
13.5~16
|
0.55
|
0.5≤d≤1
|
9~11
|
0.5
|
MAI-S6
|
3<d≤6
|
48~75
|
0.55
|
99.8
|
20:1
|
7.0
|
4850*3670*2750
|
3500
|
1<d≤3
|
17.5~21
|
0.55
|
0.5≤d≤1
|
10.5~14
|
0.5
|
The above index is based on 15% impurity content quartz as an example,The specific index varies with the material and impurity content.
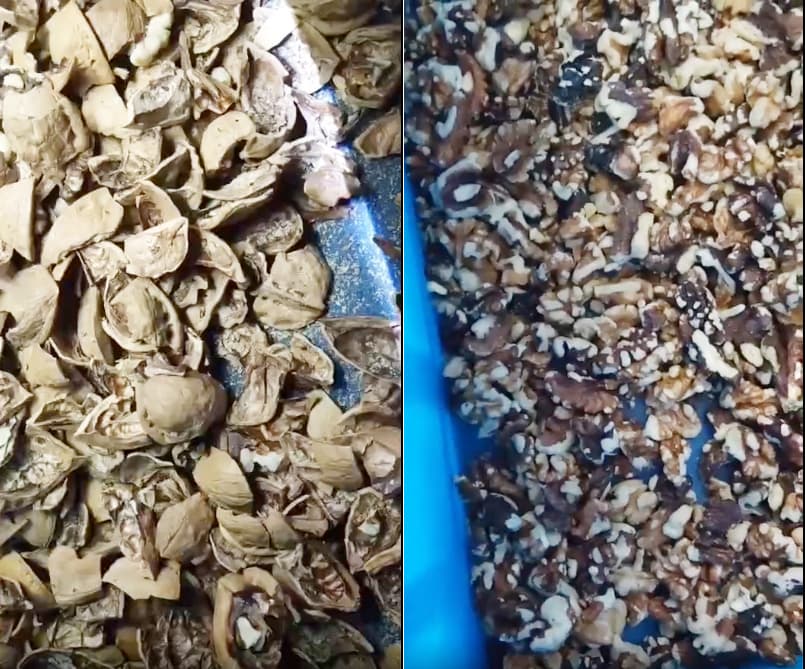
Applicable sorting ore materials
Talc, wollastonite ,calcite, quartzite
Fluorite, Potassium feldspar, Magnesite, Lithium pyroxene
Phosphate ore, Gold ore, High crystal silicon, oxide copper ore
Barite, bauxite ,lead-zinc ore and fluorite, barite lead-zinc ore
Not limited to the above ores, eye visible distinctions are applicable to AI sorting machines!
A wide range of sorting materials, can be applied to nuts, grains and cereals, Chinese herbs, dehydrated food and other materials sorting.